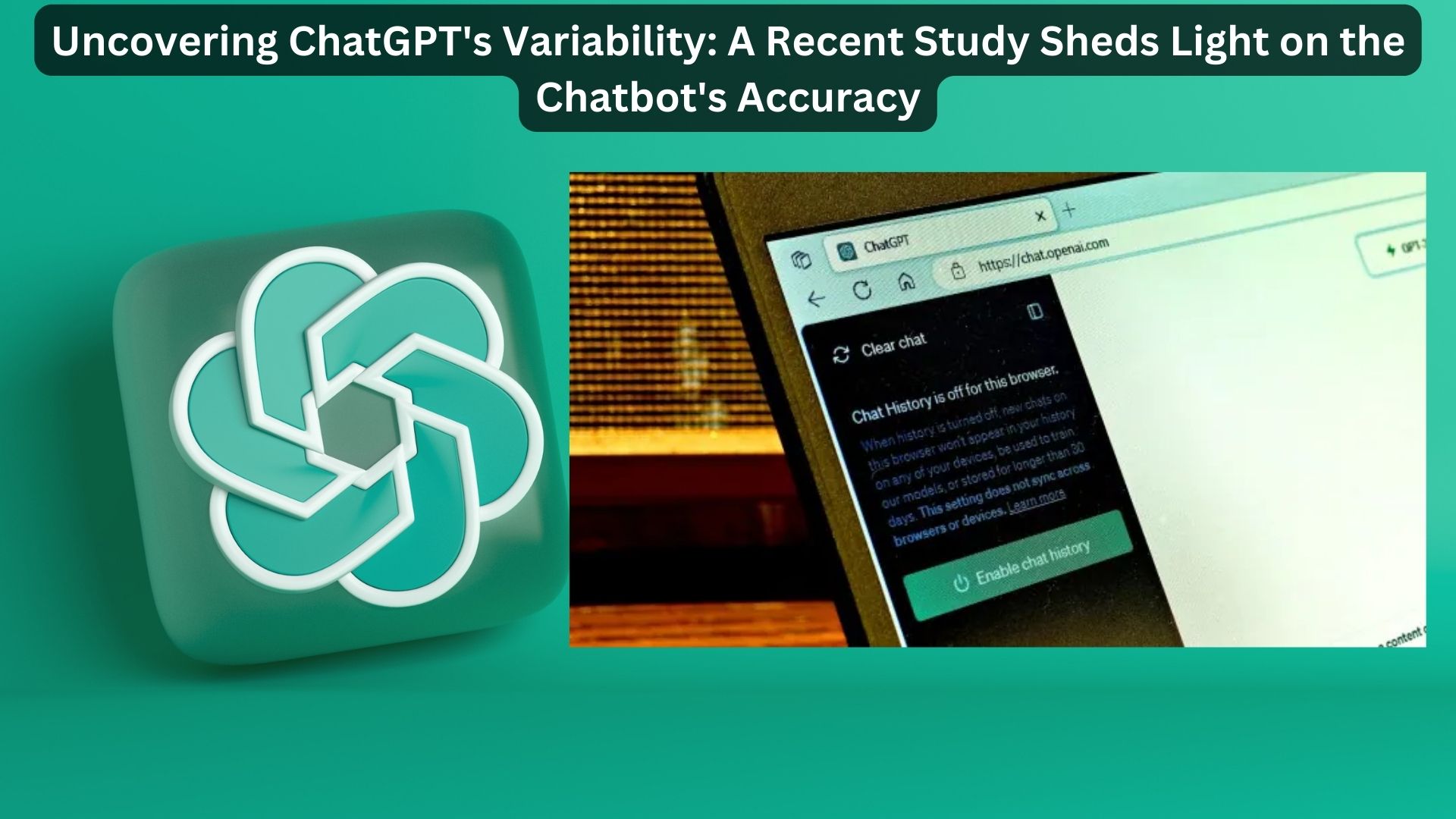
A recent study from Stanford University has raised concerns about the consistency and accuracy of ChatGPT, a popular generative artificial intelligence (AI) chatbot.
The Stanford research team conducted a comprehensive evaluation of ChatGPT’s performance across various tasks, including mathematics problem-solving, addressing sensitive inquiries, writing software code, and visual reasoning.
What were the key findings of the study?
The study revealed significant fluctuations in ChatGPT’s capabilities over time. Currently, ChatGPT is available in two versions: the free GPT-3.5 model and the paid GPT-4 model, which is promoted as being smarter and faster.
In the realm of mathematics, GPT-4 initially demonstrated impressive problem-solving abilities in March, correctly identifying prime numbers 97.6% of the time. However, just three months later, its accuracy plummeted to a mere 2.4%. Interestingly, GPT-3.5 exhibited an opposite trend, improving from 7.4% accuracy to an impressive 86.8% accuracy during the same period.
Similar fluctuations were observed when ChatGPT was engaged in tasks such as writing code and visual reasoning. James Zou, a Stanford computer science professor involved in the study, remarked, “When we fine-tune a large language model to enhance its performance in specific tasks, unintended consequences may emerge, potentially compromising its performance in other tasks. There are intricate interdependencies in how the model responds, which can lead to the deteriorating behaviors we observed.”
Researchers argue that these findings may not necessarily reflect ChatGPT’s inherent accuracy but rather highlight the unintended repercussions of fine-tuning the model. Essentially, optimizing one aspect of the model can negatively impact other tasks.
The reasons behind these fluctuations are challenging to pinpoint because ChatGPT’s inner workings remain undisclosed, and its code is not open-source. Over time, researchers noticed that ChatGPT’s responses not only became less accurate but also ceased to provide explanations for its reasoning. For instance, if you asked ChatGPT to explain its methodology for solving a mathematical problem, it would often skip this step, according to the researchers.
In the context of sensitive topics, both GPT-4 and GPT-3.5 adopted a cautious stance, citing that certain prompts conveyed discriminatory ideas. By June, ChatGPT had become entirely unresponsive to such inquiries. ChatGPT’s elusive operational nature makes it challenging to comprehensively study and assess its performance. This study underscores the necessity for ongoing monitoring and evaluation of performance fluctuations in large language models (LLMs), which power tools like ChatGPT.
Also Read